GPT-3 and Chat GPT in Insurance: What you should know about the AI chatbot
Since the beginning of December, the GPT-3 or Chat GPT language model has been causing a stir again. We have tested Chat GPT intensively and want to clarify crucial questions: What is GPT-3? What is Chat GPT? What are the possibilities of using Chat GPT in the insurance industry? And what are the challenges of using Chat GPT for speech processing in the insurance industry?
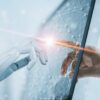
What is GPT-3?
GPT-3 is a neural network model for machine learning and natural language processing developed by OpenAI. It is one of the most powerful models in the field and has been proven in various applications such as machine writing, translation, and dialog systems. GPT-3 is capable of generating human-like text and can also be used for other tasks such as question-answering systems, document classification, and summaries.
What is Chat GPT?
Chat GPT is an application based on OpenAI’s GPT-3 model that is a chatbot system. It is designed to conduct human-like dialogues and can be used for various applications such as customer support, marketing, and entertainment. Chat GPT uses the GPT-3 model to analyze user input and generate responses based on it.
What are the opportunities for using Chat GPT in the insurance industry?
There are many ways Chat GPT or other chatbots can be used in the insurance industry. Some examples of how chatbots can be used in the insurance industry are:
- Contract management: chatbots could be used to help customers manage their insurance contracts, such as requesting information about contract content or processing contract changes.
- Claims management: Chatbots could be used to receive and process claims notifications from customers, for example by assisting customers in submitting claims notifications or automatically forwarding claims notifications to the appropriate contact person.
- Customer advice: Chatbots could be used to help customers find the right insurance, for example by providing information about different insurance products or advising customers on choosing the right insurance.
- Customer support: chatbots could be used to handle and respond to customer inquiries, for example, by helping customers find information or automatically generating answers to frequently asked questions.
What are the challenges of using Chat GPT for insurance speech processing?
There are several challenges to consider when using Chat GPT for insurance speech processing. These include:
- Technical language: the insurance industry has its own technical language that can be difficult for those outside the industry to understand. Chat GPT must be able to understand and process this jargon to deliver accurate results.
- Jurisdiction: the insurance industry is subject to numerous laws and regulations that impact the language and content of documents. Chat GPT must be able to understand and accommodate these regulations to deliver accurate results.
- Different document types: In the insurance industry, there are different document types such as applications, policies, and claims that contain different information and are structured differently. Chat GPT must be able to understand and account for these differences to provide accurate results.
- Incomplete or inconsistent data: In the insurance industry, there is sometimes incomplete or inconsistent data that makes it difficult to understand documents. Chat GPT must be able to handle such incompleteness and inconsistencies and still deliver accurate results.
Chat GPT on test
Do you believe us when we say that Chat GPT has machine generated all the answers to the above questions? Prove us wrong 🙂
Indeed it did. We simply asked the model the questions and got exactly those answers or parts of the answers. Sounds impressive at first – right?
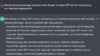
But how good is Chat GPT really and what of the developments can actually be applied in the insurance industry?
We tested the chatbot with some inputs and questions. In addition to the questions and answers listed above, the model was able to formulate short stories and articles on any topic for us upon request. The amazing thing: Contextual knowledge is required for generation, which the model is obviously equipped with. Where the answers surprise on the one hand, they can also confuse on the other. The truth of the machine-generated answers can only be verified by explicit knowledge about a subject. Sources or data that can be used to explain the answers are not provided. We also found that the same question did not always yield the same answer. Answers were close in content, but sometimes differed in wording and reasoning.
Chat GPT and the insurance language
Insurance-specific training
A language model is only as good as the data used to train it. You can think of this process as when a young child learns to speak. The older a child gets, the more words he or she learns. The child’s environment also plays a role. If a child grows up in a household with two lawyers and has mostly contact with other lawyers, he or she will certainly know some legal terminology, unlike other children.
In terms of a language model, this means: The more specific the data set used to train a model, the better results can be expected in a given domain. So what does this mean for the insurance industry or insurance language? As in any industry, there are some words that insurance professionals need to know. When a non-insurance professional tries to understand a general insurance term, they may reach their limits.
Therefore, if a language model is to be able to understand appropriate insurance vocabulary such as indemnity limit, zillmerization, or waiting period, it must be trained with appropriate texts. Since there are also line-specific terms in addition to insurance-specific terms, additional line-specific fine-tuning can be useful. However, the language model alone is not responsible for successful use.
Model adjustments required
Chat GPT is trained on a general document corpus and can only relate its answers to its trained knowledge. In this case, it is not possible to consider individual documents or facts without model adaptations. In order to implement use cases relevant to the insurance industry, we believe that fine-tuning is not only possible, but mandatory. For example, if you want to automatically analyze and/or compare insurance texts, you have to build an entire process line with OCR and data preprocessing in addition to a model trained on insurance language. In many cases, contextualization is crucial for insurance texts. For this, it is important to make heterogeneous text formatting processable: Among other things, text sections must be contextualized with headings or enumerations must be resolved.
Conclusion
In summary, Chat GPT is not readily applicable in the insurance industry. There are numerous technical, legal and ethical challenges that must be evaluated on a case-by-case basis. The use of language models is in itself quite useful and purposeful in various use cases. We would be happy to discuss with you the possible applications of Natural Language Processing. Please do not hesitate to contact us.
Authors
Lukas Pulß – Management Consultant
As an experienced IT consultant and insurance expert, Lukas’ expertise is primarily characterized by his comprehensive technical and industry knowledge as well as his experience in the entire lifecycle of IT projects. As a product owner, he forms the technical bracket over the AI topics in the Insurance business unit.
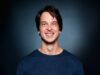
Maximilian Lorenz – Data Scientist
During his mathematics studies at the University of Würzburg, Maximilian Lorenz was already involved with neural networks and the use of machine learning algorithms. As a Data Scientist, he supervises AI projects for international customers from the insurance industry.